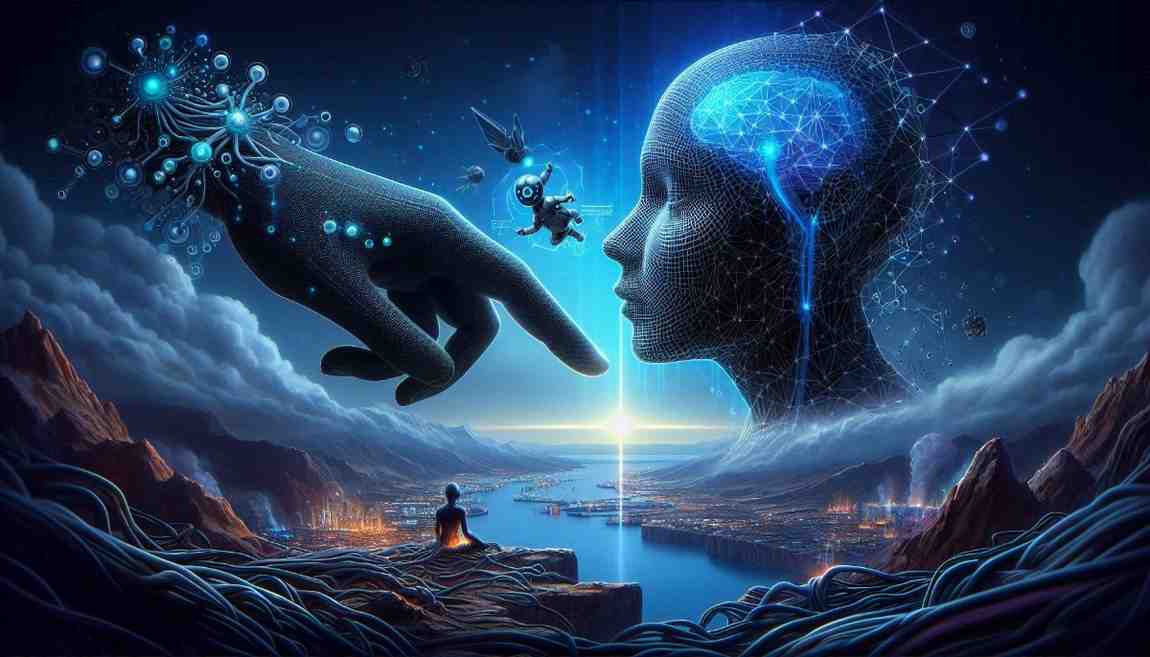
The Quiet Revolution of Edge AI: Bringing Intelligence Closer to the Source
When we talk about the future of ICT, the spotlight often shines on cloud computing, 5G, or AI breakthroughs in massive data centres. But there’s a less flashy yet profoundly transformative trend quietly reshaping how devices think and interact: Edge AI.
Edge AI refers to the deployment of artificial intelligence algorithms directly on devices at the “edge” of the network—think smartphones, IoT sensors, industrial machines, or even smart cameras—instead of relying solely on centralized cloud servers. This means data is processed locally, near where it’s generated, rather than being sent back and forth to distant data centres.
Why Does Edge AI Matter?
Processing AI locally offers several compelling advantages:
Latency Reduction: For applications like autonomous drones or real-time industrial monitoring, milliseconds matter. Edge AI eliminates the delay caused by transmitting data to the cloud, enabling instant decision-making.
Bandwidth Efficiency: Sending huge volumes of raw data to the cloud can clog networks and increase costs. Edge AI processes data on-site, transmitting only relevant insights, saving bandwidth.
Privacy and Security: Sensitive data—such as personal health metrics or proprietary industrial information—can be analysed locally without exposing it to external servers, reducing risk.
Real-World Applications You Might Not Expect
Edge AI is making waves in some fascinating, less-discussed areas:
Agriculture: Smart sensors in fields analyse soil moisture, crop health, and pest presence in real-time, enabling precision farming without constant cloud connectivity.
Wildlife Conservation: Remote cameras equipped with Edge AI identify and track endangered species or detect poachers in real-time, even in areas with limited internet access.
Manufacturing Quality Control: Edge AI-powered cameras inspect products on assembly lines instantly, catching defects faster than human inspectors or cloud-based systems could.
The Challenges on the Edge
Despite its promise, Edge AI faces unique hurdles:
Hardware Constraints: Edge devices often have limited processing power, memory, and battery life compared to cloud servers. Designing efficient AI models that fit these constraints is a major engineering challenge.
Model Updates: Keeping AI models up to date across millions of distributed devices requires robust, secure mechanisms for remote updates.
Security Risks: While local processing enhances privacy, edge devices can be physically accessible and vulnerable to tampering, demanding strong security protocols.
The Future: Hybrid Intelligence
The future of AI in ICT likely lies in a hybrid approach, where edge and cloud AI complement each other. Edge AI handles immediate, privacy-sensitive tasks, while cloud AI manages heavy-duty training, analytics, and coordination across devices.
This balance enables smarter, faster, and more secure systems that can operate reliably even with intermittent connectivity—a crucial factor as the Internet of Things continues to explode.
Edge AI may not dominate headlines like some other ICT trends, but its impact is quietly profound. By bringing intelligence closer to the source, it’s enabling a new generation of applications that are faster, more private, and more efficient shaping the future of technology in subtle yet powerful ways.
References
- Shi, Weisong, et al. “Edge Computing: Vision and Challenges.” IEEE Internet of Things Journal, 2016.
- Satyanarayanan, Mahadev. “The Emergence of Edge Computing.” Computer, 2017.
- Lane, Nicholas D., et al. “DeepX: A Software Accelerator for Low-Power Deep Learning Inference on Mobile Devices.” IPSN, 2016.
- Zhang, Kai, et al. “Edge AI: On-Demand Accelerating Deep Neural Network Inference via Edge Computing.” IEEE Transactions on Wireless Communications, 2020.